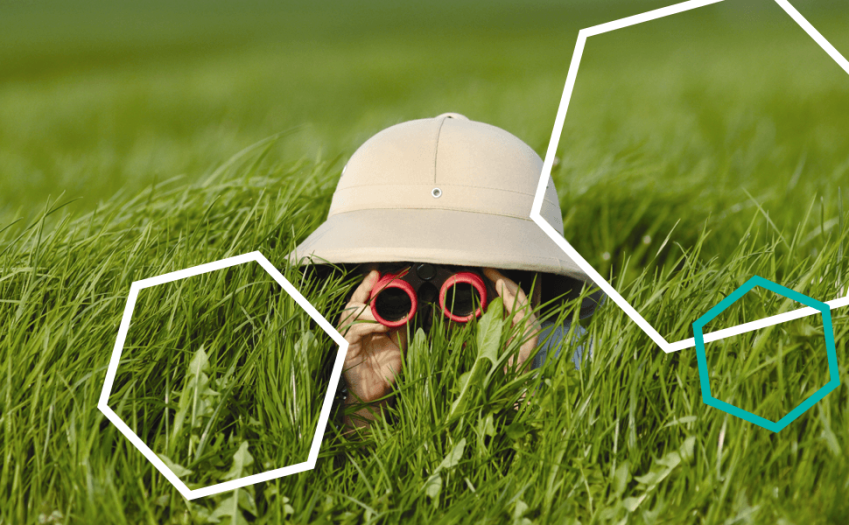
Early encounters with speech analytics transcriptions might quickly lead you to the conclusion that this technology isn’t all it’s cracked up to be.
Words are misrecognised, a whole bunch are replaced with the funny star symbol, in fact it doesn’t even seem to make much sense. How am I supposed to analyse this?
In reality you may not be far from the truth! However, despite this, it actually doesn’t matter as much as it first appears. The speech recognition task on a recorded call is very hard. The audio quality on the phone line is frequently relatively poor, there is often background noise on both the caller and advisor side, and callers frequently mumble, stumble and fumble.
In this situation, it’s often the smaller and phonetically confusable words that suffer the most. The prepositions (of, to, by, etc…) and conjunctions (and, but) may be missed entirely, numbers may be missed out or incorrect, addresses may just be nonsense. However, in the main, these aren’t the important terms for speech analytics. Speech analytics is about discovering the key sentiment behind a customer contact, and to achieve this we need good accuracy across the content words and phrases. Thankfully, in the main, these are often longer and more phonetically distinct. Think about using speech analytics to check for compliance, we are interested in terms such as “Financial Conduct Authority”, “non-advised offering”; long words with unique phonetic characteristics.
We can also exploit another useful characteristic of human language – its tendency to repetition and redundancy. Human languages evolved to achieve data transmission in a noisy and uncertain medium between low fidelity transceivers. Consequently we repeat and rephrase, a lot. This is especially useful in the speech analytics setting as callers will repeat the main reason for calling, so if the recogniser gets it wrong once or twice it may not matter.
It’s common in computational linguistics to represent documents, transcripts or even web pages as a ‘bag of words’. From this view, the high level structures such as sentences and paragraphs and – to some extent – even word order, is discarded. The task of a document classifier is to determine the purpose of the bag by looking at the occurrence of certain key words or phrases from within the bag. A similar analogy makes sense for speech analytics. Although the transcript may not be perfect, viewed as a bag of key terms and phrases, we start to see how we can get good classification and analysis results without perfect recognition.
All this is not to say the speech recognition isn’t important. Clearly it’s the foundation of the speech analytics work flow and needs to give decent results. So if key content words and phrases are consistently misrecognised, we still need to use tuning and retraining techniques available to address this.
The takeaway here is that it’s important to focus on the metrics that make the most sense for measuring speech analytics performance. In reality, word error rate isn’t one of them. Technically we should be looking at the precision and recall of the categories we’re building. Operationally we should be looking at the KPIs related to our business issues, be that AHT, number of complaints or call volumes and the benefit the speech analytics process is having on these. Find out more about Speech Analytics.